Percentage Change in Non-Linear Modeling: Going Beyond the Basics
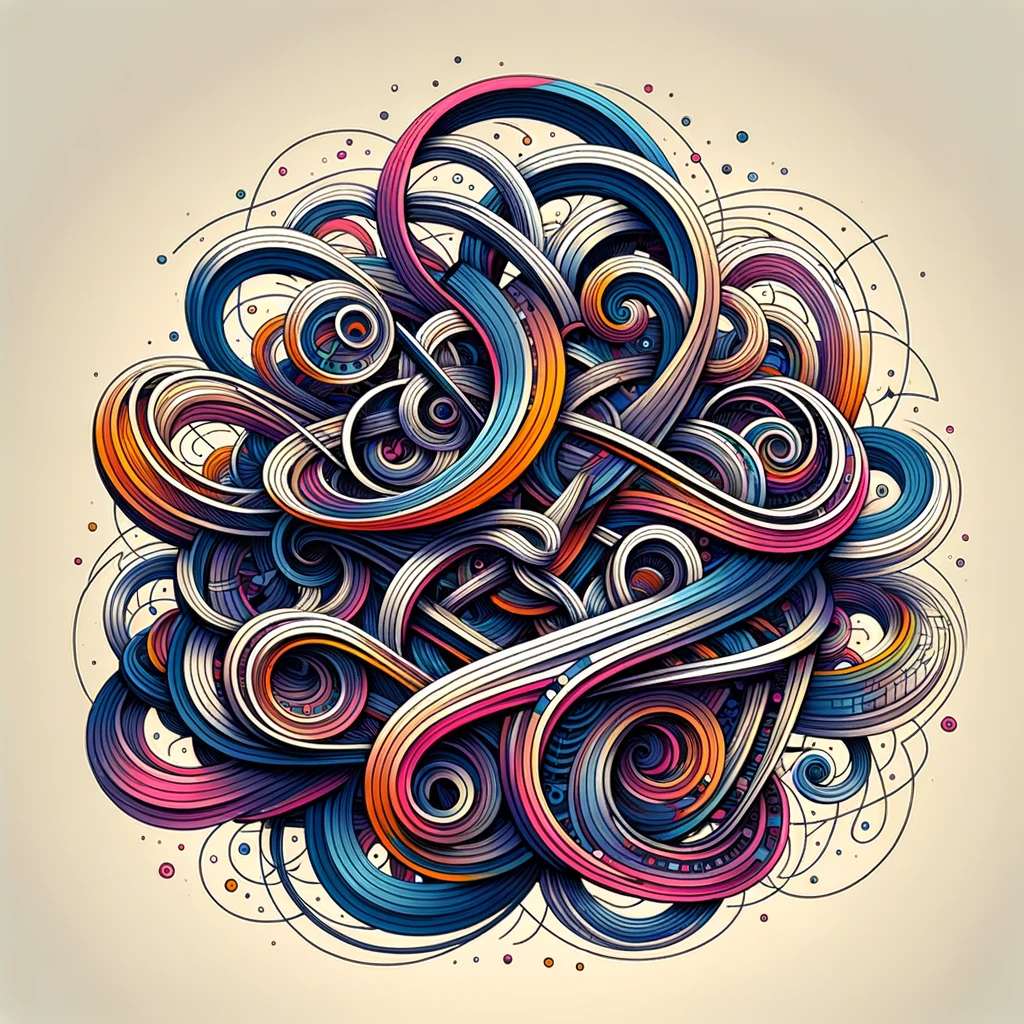
Non-linear modeling has become increasingly important in various fields such as finance, economics, and biology. While understanding the basics of non-linear models is crucial, it is equally important to explore the nuances and complexities of these models. One such aspect that is often overlooked is the percentage change in non-linear modeling.
Percentage change is a measure of how much a variable has changed in relation to its original value. In non-linear modeling, percentage change can provide insights into the behavior of the model and its variables. However, interpreting percentage change in non-linear models can be challenging, as the relationship between variables is not always straightforward.
In this article, we will explore the concept of percentage change in non-linear modeling and go beyond the basics. We will discuss the challenges of interpreting percentage change in non-linear models and explore some techniques to overcome these challenges. By the end of this article, readers will have a better understanding of how to use percentage change in non-linear modeling and how it can provide valuable insights.
Key Takeaways
- Non-linear modeling is a complex field that requires a deep understanding of its nuances and complexities.
- Percentage change is an important measure in non-linear modeling that can provide valuable insights into the behavior of the model and its variables.
- Interpreting percentage change in non-linear models can be challenging, but there are techniques that can help overcome these challenges.
Understanding Non-Linear Models
Non-linear models are an essential tool for modeling complex systems where the relationship between the input variables and the output variable is not linear. Non-linear models are widely used in various fields, including engineering, physics, economics, and finance.
Characteristics of Non-Linear Models
Non-linear models have some unique characteristics that differentiate them from linear models. First, non-linear models are more complex and challenging to estimate. Second, non-linear models have a more significant number of parameters than linear models, which means that they require more data to estimate accurately. Third, non-linear models are sensitive to the initial values of the parameters, which means that the estimation process can be time-consuming and computationally intensive.
Importance of Non-Linear Models
Non-linear models are essential in many areas of research and practice because they can capture complex relationships between variables that cannot be modeled using linear models. For example, non-linear models are widely used in finance to model the behavior of stock prices, interest rates, and exchange rates. Non-linear models are also used in epidemiology to model the spread of infectious diseases, in ecology to model population dynamics, and in physics to model the behavior of complex systems.
In conclusion, non-linear models are a powerful tool for modeling complex systems where the relationship between the input variables and the output variable is not linear. Non-linear models have unique characteristics, and they are essential in many areas of research and practice.
Exploring Percentage Change
Concept of Percentage Change
Percentage change is a measure of the difference between two values, expressed as a percentage of the original value. It is a useful tool for understanding changes over time or comparing different sets of data. In non-linear modeling, percentage change can be particularly useful for analyzing complex relationships between variables.
One way to calculate percentage change is to subtract the original value from the new value, divide that difference by the original value, and then multiply by 100. For example, if the original value is 100 and the new value is 120, the percentage change would be (120-100)/100 x 100 = 20%.
Application in Non-Linear Models
In non-linear modeling, percentage change can be used to analyze relationships that are not linear or proportional. For example, if an increase in one variable leads to a much larger increase in another variable, the relationship between the two may be non-linear. By calculating percentage change, researchers can better understand the nature of these relationships and make more accurate predictions.
One common application of percentage change in non-linear modeling is in financial forecasting. By analyzing percentage changes in stock prices, interest rates, and other financial indicators, analysts can make more informed decisions about investment strategies and risk management.
Overall, exploring percentage change in non-linear modeling can provide valuable insights into complex relationships between variables. By using this tool, researchers and analysts can make more accurate predictions and better understand the factors that drive change over time.
Beyond the Basics
Non-linear modeling is a powerful tool for analyzing complex data sets. One of the key challenges in non-linear modeling is determining the percentage change in the response variable for a given change in the predictor variable. While basic techniques for calculating percentage change are well known, advanced techniques can provide more accurate and nuanced results. In this section, we will explore some advanced techniques for calculating percentage change in non-linear modeling.
Advanced Techniques
One advanced technique for calculating percentage change is to use the log-transformed response variable. By taking the natural logarithm of the response variable, the relationship between the predictor and response variables becomes linearized. This allows for more accurate estimation of percentage change, particularly for large changes in the predictor variable.
Another advanced technique is to use the partial derivative of the response variable with respect to the predictor variable. This technique is particularly useful when the non-linear model includes multiple predictor variables. By taking the partial derivative, the effect of each predictor variable on the response variable can be isolated and analyzed.
Real-World Examples
To illustrate the usefulness of these advanced techniques, consider a study of the effect of temperature on the growth rate of a particular species of plant. A non-linear model is used to estimate the growth rate as a function of temperature. By using the log-transformed response variable, the percentage change in growth rate can be accurately estimated for a given change in temperature.
In another study, a non-linear model is used to estimate the effect of various environmental factors on the mortality rate of a particular species of animal. By using the partial derivative technique, the effect of each environmental factor on the mortality rate can be analyzed separately, allowing for a more nuanced understanding of the complex interactions between the different factors.
In conclusion, advanced techniques for calculating percentage change in non-linear modeling can provide more accurate and nuanced results than basic techniques. These techniques, such as the log-transformed response variable and partial derivative, can be particularly useful when analyzing complex data sets with multiple predictor variables.