Predictive Modelling: Integrating Percentage Change Techniques for Accurate Forecasting
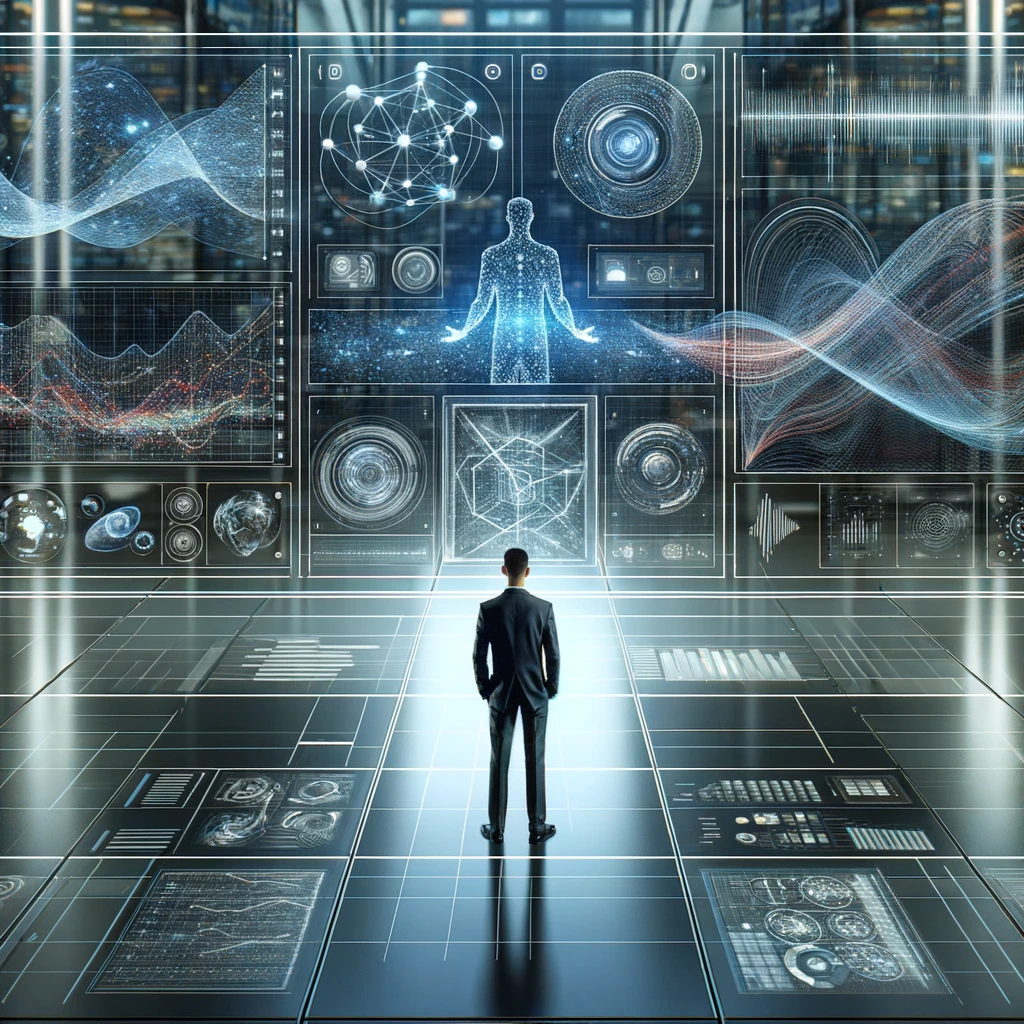
Predictive modelling is a powerful tool that helps businesses and organizations make informed decisions based on data analysis. By using a variety of statistical techniques, predictive modelling can forecast future trends and outcomes with a high degree of accuracy. One such technique is percentage change analysis, which can be integrated into predictive modelling to provide even more valuable insights.
Percentage change analysis involves comparing the percentage difference between two values over a given period of time. This technique is commonly used in finance and economics to measure growth rates and inflation. When integrated into predictive modelling, percentage change analysis can help identify trends and patterns that might not be apparent from raw data alone. For example, if a company wants to forecast sales for the next quarter, percentage change analysis can be used to identify seasonal trends and adjust the forecast accordingly.
Integrating percentage change techniques into predictive modelling requires a deep understanding of both statistical analysis and the specific business context. There are many different methods for calculating percentage change, and choosing the right one depends on the type of data being analyzed and the goals of the analysis. However, when done correctly, integrating percentage change techniques can greatly enhance the accuracy and usefulness of predictive modelling.
Key Takeaways
- Predictive modelling is a powerful tool for making informed decisions based on data analysis.
- Percentage change analysis is a technique commonly used in finance and economics to measure growth rates and inflation.
- Integrating percentage change techniques into predictive modelling can greatly enhance the accuracy and usefulness of the analysis.
Predictive Modelling: An Overview
Definition and Importance
Predictive modelling is a process of using statistical and machine learning algorithms to analyze data and make predictions about future events or behaviors. It is a powerful tool that can help businesses and organizations make informed decisions and improve their operations.
Predictive modelling is important because it allows businesses to anticipate future trends, identify potential risks, and make proactive decisions. By using predictive modelling, businesses can gain a competitive advantage by being able to react quickly to changes in the market.
Key Components
There are several key components of predictive modelling, including data collection, data preparation, model building, and model deployment.
Data collection involves gathering relevant data from various sources, such as customer data, sales data, and market data. Data preparation involves cleaning, transforming, and organizing the data so that it can be used for analysis.
Model building involves selecting the appropriate statistical or machine learning algorithm and training the model on the prepared data. The goal is to create a model that accurately predicts future events or behaviors.
Model deployment involves integrating the model into the business operations and using it to make predictions and inform decision-making.
Overall, predictive modelling is a powerful tool that can help businesses and organizations make informed decisions and gain a competitive advantage in the market. By understanding the key components of predictive modelling, businesses can effectively integrate it into their operations and reap the benefits of accurate predictions and proactive decision-making.
Percentage Change Techniques: A Closer Look
Understanding Percentage Change
Percentage change is a widely used technique in finance and economics to analyze trends and fluctuations in data. It is a simple calculation that expresses the difference between two values as a percentage of the original value.
For example, if the price of a stock was $100 yesterday and it is $110 today, the percentage change in price would be (110-100)/100 = 10%. This technique is useful for comparing changes in values of different magnitudes, as it normalizes the data to a common scale.
Role in Predictive Modelling
Percentage change techniques play a crucial role in predictive modelling, especially in time series analysis. By analyzing historical data and identifying patterns in percentage changes, predictive models can be developed to forecast future values.
For instance, if a company wants to predict the sales of a product for the next quarter, they can use percentage change techniques to analyze the sales data of the previous quarters and identify any trends or seasonality. This information can then be used to develop a predictive model that takes into account the percentage changes in sales over time.
In conclusion, percentage change techniques are a powerful tool in predictive modelling that can help businesses make informed decisions based on historical data. By understanding the basics of percentage change and its role in predictive modelling, businesses can develop accurate and effective models to forecast future trends and make informed decisions.
Integration of Percentage Change Techniques into Predictive Modelling
Predictive modelling is an essential tool for businesses and organizations to forecast future trends and make informed decisions. One of the techniques used in predictive modelling is percentage change analysis. The integration of percentage change techniques into predictive modelling has proven to be an effective approach for improving forecasting accuracy.
Practical Approaches
There are various practical approaches to integrating percentage change techniques into predictive modelling. One common approach is to use historical data to calculate the percentage change in a variable over time. This information can then be used to create a predictive model that takes into account the percentage change of the variable.
Another approach is to use percentage change as a feature in the predictive model. This involves including the percentage change of a variable as an input in the model. This approach can improve the accuracy of the model by taking into account the trend of the variable over time.
Benefits and Challenges
The integration of percentage change techniques into predictive modelling has several benefits. Firstly, it can improve the accuracy of the model by taking into account the trend of the variable over time. This can lead to better decision-making by businesses and organizations.
Secondly, percentage change techniques can help identify trends and patterns in the data that may not be apparent through other methods. This can lead to new insights and opportunities for businesses and organizations.
However, there are also challenges associated with the integration of percentage change techniques into predictive modelling. One challenge is the need for accurate and reliable historical data. Without this data, the percentage change analysis may not be effective.
Another challenge is the need for expertise in statistical analysis and predictive modelling. Businesses and organizations may need to invest in training or hiring experts to effectively integrate percentage change techniques into their predictive modelling processes.
In conclusion, the integration of percentage change techniques into predictive modelling can be a valuable tool for businesses and organizations. While there are challenges associated with this approach, the benefits of improved accuracy and new insights make it worth considering for predictive modelling processes.
Conclusion
In conclusion, integrating percentage change techniques into predictive modelling can greatly enhance the accuracy and reliability of the model’s predictions. By using percentage change, analysts can account for the effects of inflation, seasonality, and other factors that may affect the data over time.
One advantage of using percentage change is that it can help to normalize the data, making it easier to compare across different time periods or variables. This can be particularly useful when analyzing trends or patterns in the data.
Another benefit of using percentage change is that it can help to identify outliers or anomalies in the data. By looking at the percentage change over time, analysts can quickly identify any unusual fluctuations or trends that may require further investigation.
Overall, integrating percentage change techniques into predictive modelling can help to improve the accuracy and reliability of the model’s predictions. By taking into account the effects of inflation, seasonality, and other factors, analysts can ensure that their predictions are more accurate and reliable, helping to inform better decision-making.